Ontology Mapping
Empowering insights through unified client data mapping.
Faethm by Pearson's occupation mapping is transforming how organisations leverage skills and jobs data. It provides organisations with a comprehensive understanding of their workforce by seamlessly bridging the gap between disparate data sources.
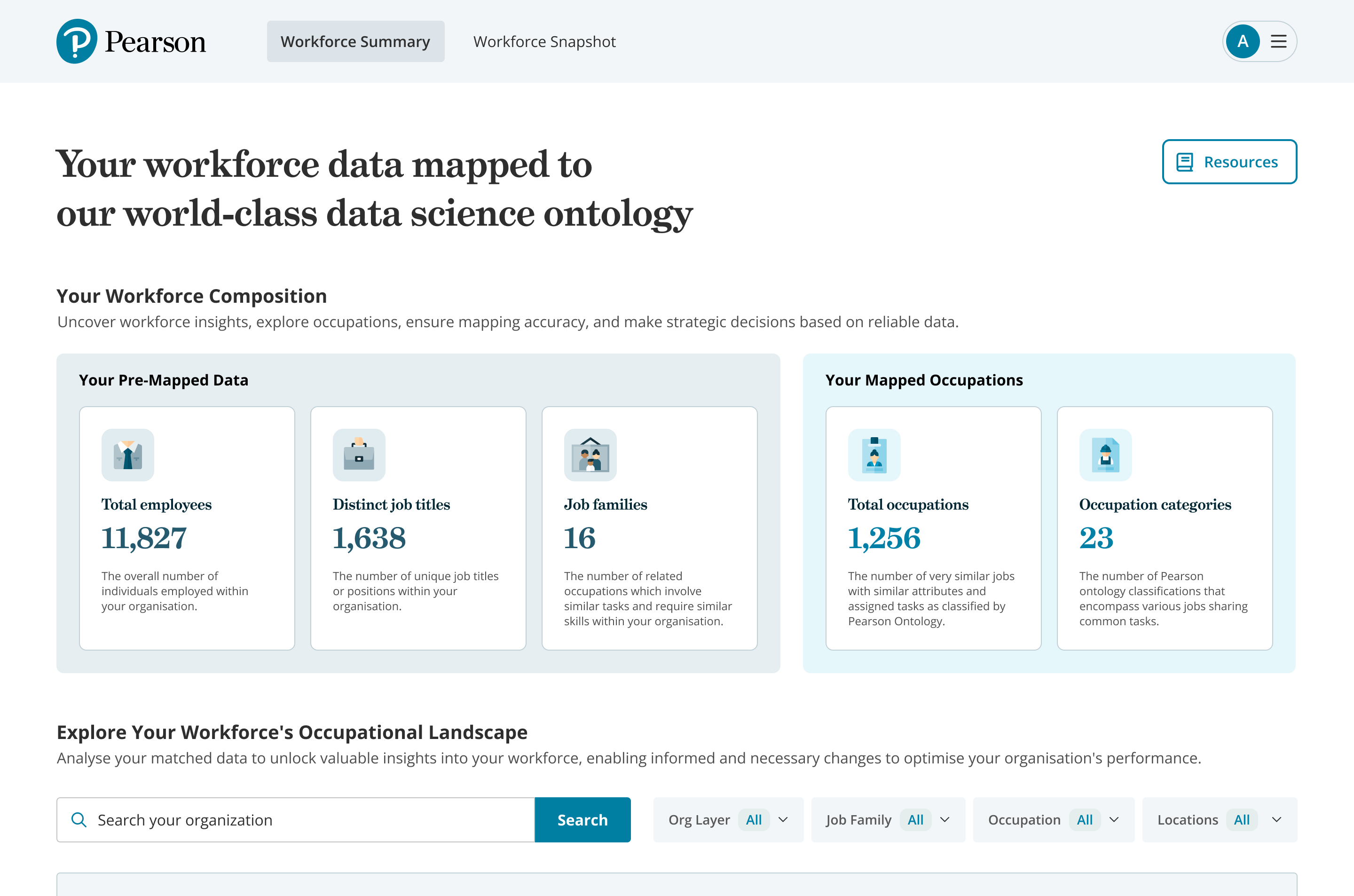
The Problem
A HR manager responsible for inputting organisational data into our system faces the cumbersome and time-consuming task of manual data entry and validation. This hinders their ability to fully utilise the platform's value until this task is completed, which can take anywhere from 90 to 120 business days.
“Seamless customer data onboarding benefits everyone involved— by reducing the manual labor required for wrangling data, it leads to a faster time to value for customers because teams spend less time cleaning data and more time on revenue-generating work.”
– Osmos.io (https://www.osmos.io/guides/customer-data-onboarding)User Research
Insights from internal and external interviews were gathered to understand the organisation's internal processes, goals, challenges, as well as end users' experiences, challenges, and expectations. These insights guided the discovery and development process, ensuring the end product addressed pain points, streamlined operations, and optimised user experiences.
"Theres been organisations who've had a validation file sitting with them for a good six months."– Customer Insights Manager, Pearson
"It can be a little confusing for people who see the ontology for the first time, especially with different levels."– Customer Insights Manager, Pearson
"I think the main issue we have, is anytime you do anything manually its subject to an error."– Customer Insights Manager, Pearson
Research Findings
Both internal and external research have uncovered challenges that impact our platform and end-users. These findings include:
- Users spend several hours a day manually matching job roles and troubleshooting data discrepancies, with Pearson’s ontology manager being solely responsible for manually matching the client’s job roles to the ontology.
- The validation process, done by csv file, takes an average of 90 business days due to manual job matching and low-confidence role reviews, causing significant delays in onboarding to the platform.
- Unexpected data changes by clients negatively impact platform readiness.
- Several users express difficulty in understanding the ontology, making the onboarding process challenging.
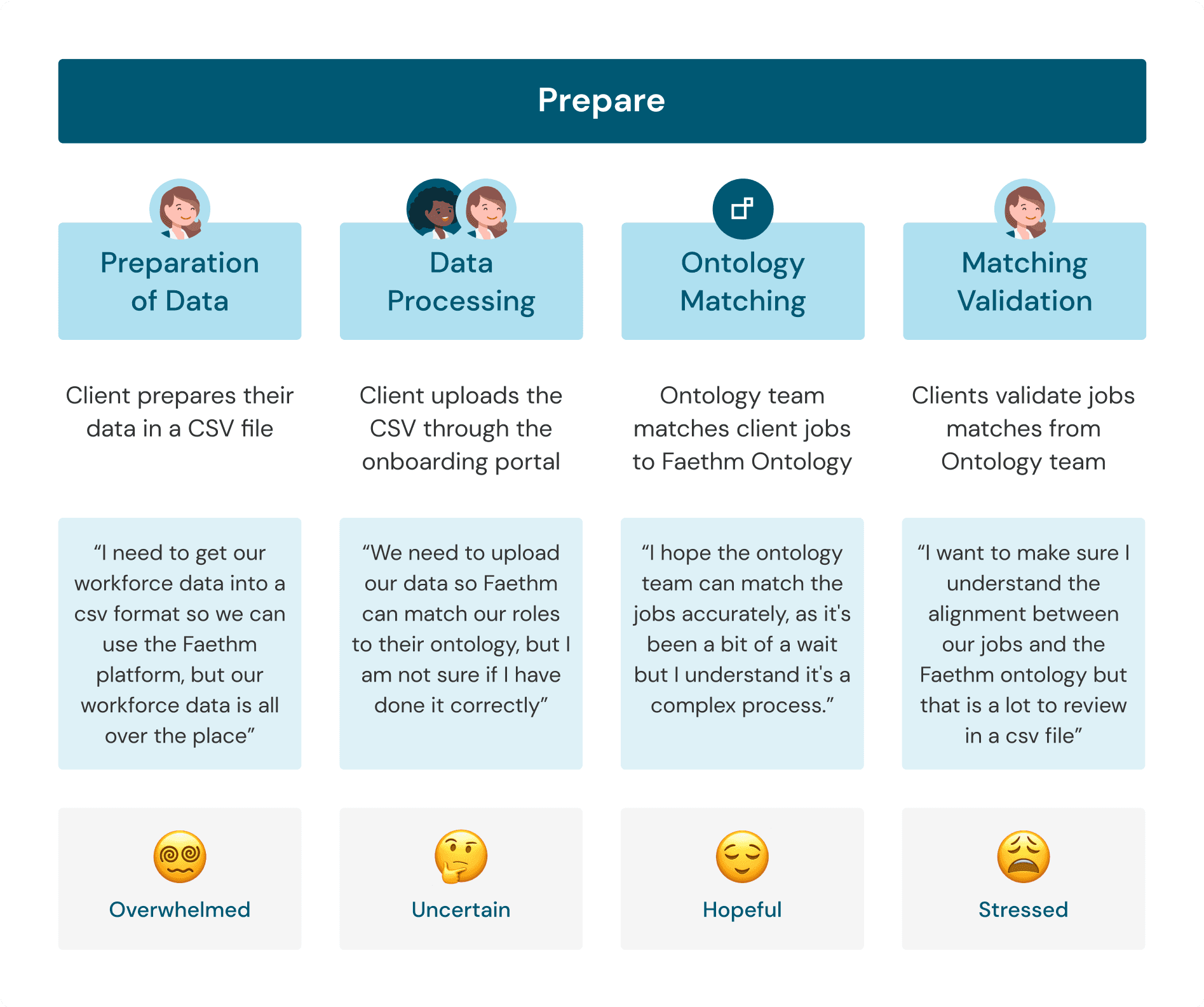
Research Insights
The research findings unveiled invaluable insights into the challenges and pain points within the data onboarding process. These insights include:
- The manual job matching process and troubleshooting of data discrepancies are resource-intensive and time-consuming for users. The heavy reliance on the ontology manager for these tasks suggests an opportunity to streamline and automate these processes to improve user efficiency and reduce operational overhead.
- The lengthy validation process, primarily caused by manual job matching and low-confidence role reviews, presents a critical bottleneck in the onboarding process. This finding highlights the need to implement a more efficient, and automated validation procedure to expedite the onboarding of users onto the platform and reduce time-to-value.
- Unforeseen data changes by clients can impact platform readiness, highlighting the importance of enhancing the platform's adaptability and data management capabilities. This finding shows the need to develop strategies to handle and accommodate such changes more effectively to ensure a smoother onboarding process for clients.
- The finding that users' struggle to grasp the ontology indicates a potential barrier to successful onboarding. This emphasises the need to improve user training and documentation regarding the ontology, as well as possibly simplifying or providing additional support for users who may not have prior experience.
User Profile
Through research, a persona named Fei Fei, representing a typical user, was developed to gain deeper insights into the needs, preferences, and behaviours of the target audience. Fei Fei's job responsibilities include creating and retaining a consistent supply of top talent, reviewing job details, and providing quarterly people analytic reporting.
We discovered that Fei Fei often had to rely on spreadsheets and manual data cleaning processes to align the company's data with the platform.
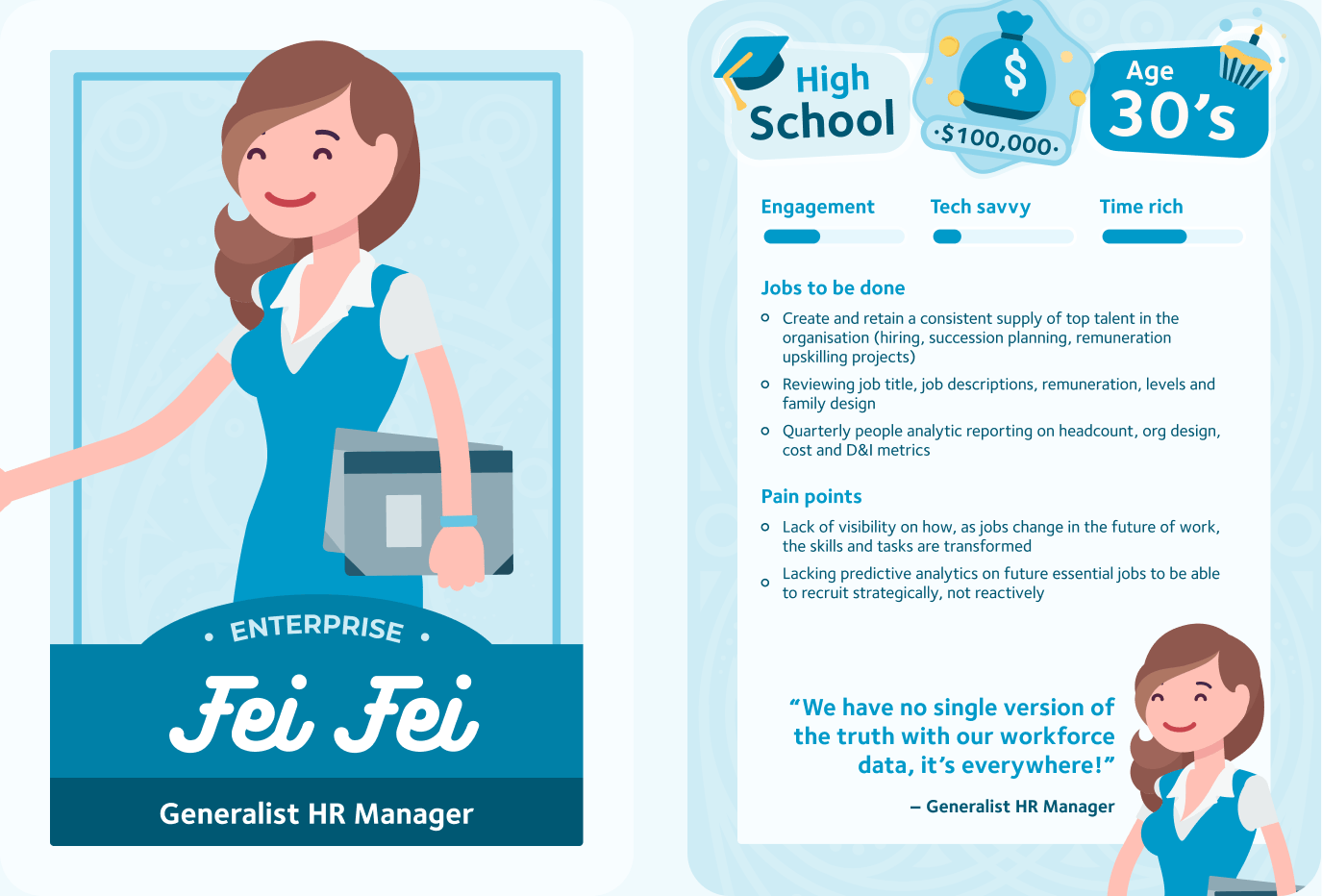
Recommendations
To improve operational efficiency and deliver a more user-friendly experience, the team ask this question:
“How might we enable clients to quickly and efficiently access insights on and get value from our platform?”
The following recommendations were proposed:
- Automated error detection: To address the finding that users spend significant time troubleshooting data discrepancies, the implementation of an automated error detection feature, could assist the company to enhance operational efficiency by reducing resource-intensive manual processing.
- Automated job matching: The validation process takes a long time due to manual processing, by introducing automated job matching through machine learning algorithms, the company could improve operational efficiency and reduce the clients time-to-value.
- Data change controls: As unexpected data changes can negatively impact platform readiness, by enforcing data change controls, the company aims to mitigate the issue and enhance data management capabilities.
- Accessible ontology framework: As users have difficulty understanding the ontology framework, by providing comprehensive documentation the company can enable users to efficiently interpret and navigate the ontology framework, while enhancing the efficiency of the customer support team.
Conceptualisation
The team embarked on a user-centred journey, driven by research, ideation, and prototyping. Throughout the project, the product underwent rigorous testing at various stages, including insightful stakeholder feedback on lo-fi prototypes. This approach ensured the creation of meaningful and impactful experiences for users.
The Solution
The ontology mapping product was designed to directly address the challenges and pain points identified through research insights. Our solution focuses on streamlining the data onboarding process, minimising manual work, enhancing data accuracy, and empowering users with comprehensive resources.
Key features that define the solution are as follows:
- Mapping validation: To address the issue of users spending excessive time manually matching job roles and troubleshooting data discrepancies, we have introduced the the edit role recommendations feature. This empowers users to review and edit job-match recommendations, enhancing data accuracy and relevance. Discrepancies in job roles can now be quickly identified and rectified within the platform, significantly reducing manual work.
- Automated job mapping: Acknowledging the prolonged validation process due to manual job matching, we have implemented an automated mapping system. This feature employs machine learning algorithms to automatically match job roles from the clients data to the ontology, reducing validation time and improving efficiency. This leads to faster onboarding and reduced time-to-value.
- Data change controls: To prevent changes to critical data fields and maintain data integrity, we have introduced robust data controls. These controls restrict user access to fields that should not be modified, ensuring data stability and platform reliability.
- Self-serve ontology framework: Recognising that some users find it challenging to understand the ontology framework, we have created a resources area on the platform where users can explore and comprehend the standardised occupation ontology through detailed explanations of the ontology classifications. This feature facilitates informed decision-making and empowers users with better understanding.
- Self-serve client resources: In line with the recommendation to improve user training and documentation, we have introduced documentation on the resources section of the platform where users have access to comprehensive documentation and FAQs, reducing the learning curve and enabling them to maximise the platform's value independently.
Summary
By addressing the challenges of data onboarding, Faethm by Pearson's Ontology Mapping revolutionises how organisations leverage skills and jobs data, providing users with a seamless and efficient experience.
For instance, HR Managers can now onboard their data in as little as a few minutes to a few hours (depending on dataset size), a substantial improvement from the previous average of 90 business days, thanks to the implementation of the automated validation processes.
This remarkable reduction in onboarding time empowers organisations to access their workforce data in a more timely manner, enabling them to make faster, data-driven decisions and enhance their overall operational agility.
The results of this project:
Client data onboarding time drastically reduced from an average of 90 business days to just a few hours.
Project Learnings
Project learnings have been instrumental in shaping our journey, underlining the significance of key practices to achieving product success. These practices included:
- Collaboration and cross-functional coordination: Effective collaboration and communication between different teams, such as ontology managers and developers, were vital for integration and alignment of processes.
- Prioritisation: Creating a strategic plan and focusing on launching a minimum viable product (MVP) helped maintain project scope, avoid distractions, and deliver a high-quality product within the desired timeframe.
- Seeking feedback early and continually: Actively involving stakeholders and users throughout the design process, seeking feedback, and testing solutions in various forms early on saved time and minimised the need for extensive rework later on.